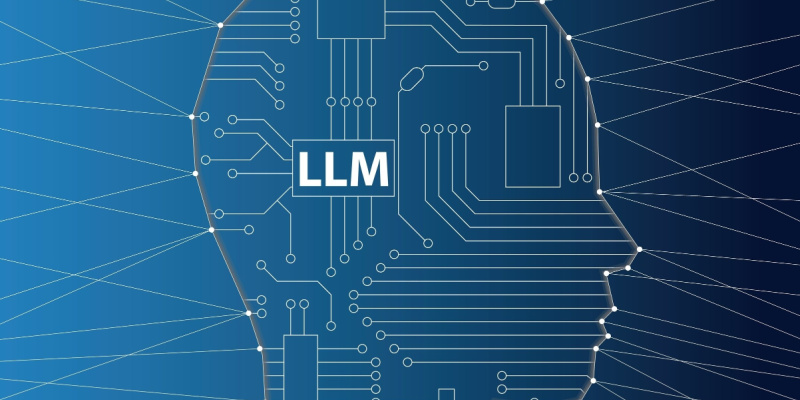
The DeepMind framework offers a breakthrough in Large Language Models’ (LLMs) reasoning by enabling self-discovery of task-intrinsic reasoning structures. This innovative approach allows LLMs to autonomously uncover reasoning modules, such as critical thinking and step-by-step analysis, to construct explicit reasoning structures, leading to improved performance in solving complex problems. The framework, developed by researchers at Google DeepMind and the University of Southern California, has shown significant enhancements in tackling challenging reasoning tasks, outperforming traditional methods by up to 32% and reducing inference computing by 10 to 40 times. Through self-discovery, LLMs can build explicit reasoning structures, enhancing their problem-solving capabilities and efficiency.
What Is LLM.
How does the Deepmind framework work.
The DeepMind framework is designed to enhance the reasoning capabilities of large language models (LLMs) by enabling them to discover and use task-intrinsic reasoning structures. This is achieved through a two-step process:
- Teaching the LLM to create a reasoning structure: The first step involves teaching the LLM to create a reasoning structure that is related to a given task and then using an appropriate reasoning module.
- Allowing the LLM to follow a path of self-discovery: The second step involves allowing the LLM to follow a path of self-discovery that leads it to a desired solution. This is done by giving the LLM the ability to use reasoning modules that have been developed through other research efforts, such as critical thinking and step-by-step analysis.
This approach allows LLMs to build explicit reasoning structures, rather than relying on reasoning conducted by others when creating their documents. The self-discovery approach has been shown to greatly improve results, consistently outperforming chain-of-thought reasoning and other current approaches by up to 32%. It also improves efficiency by reducing inference computing by 10 to 40 times.
In addition to the self-discovery approach, DeepMind also emphasizes the importance of considering the safety and ethical implications at each level of AGI development. As capabilities increase, new risks emerge, ranging from misuse and alignment issues to societal and geopolitical impacts. DeepMind proposes six guiding principles for an AGI framework, focusing on capabilities, generality and performance, cognitive and metacognitive tasks, potential, ecological validity, and path to AGI.
DeepMind’s framework also introduces a matrix framework, categorizing AI systems based on performance (Emerging, Competent, Expert, Virtuoso, Superhuman) and generality (Narrow, General). This approach allows for a nuanced understanding of where current AI systems stand and what milestones lie ahead1.DeepMind’s framework for AGI development is designed to clarify and standardize the discourse around AGI, providing a structured way to evaluate and communicate progress in AI research. It sets the stage for more focused research, clearer communication among stakeholders, and a responsible pathway towards the future of AI.